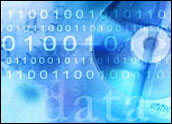
Business intelligence applications have always delighted company executives — at least as they watched simulated exercises on a sales rep’s laptop. Real-world execution, though? Until recently, that has left much to be desired.
To be sure, it is not entirely the fault of the vendor. Earlier generations of BI applications have been beset with problems that are typical to many advanced systems — particularly usability, or the lack thereof, according to Yankee Group analyst Sheryl Kingstone.
“These applications are being designed now with the line of business user in mind,” she told CRM Buyer. “That alone is a major step forward for this space.”
The end result for users is that newer generation BI applications are geared more effectively toward solving real-world business problems.
Rant Mining
Take, for example, the issue of using e-mails as part of a customer communication channel. A lot of customers shun the phone in favor of e-mail — at least, until they start to suspect their e-mails are landing on some server where they’re forever unread.
More companies would like to use the massive influx of unstructured data they receive from customers’ e-mails, Colin Shearer, senior vice president of market strategy forSPSS, told CRM Buyer. They certainly don’t want customers to walk away with the perception that their missives are not read.
“Companies know that they can determine many things from these e-mails, including valuable attitudinal data,” he said.
Enter a relatively new application called “text mining,” or “rant mining,” as it is sometimes dubbed. As the name implies, text mining parses sentences to determine the emotions behind them, using natural language processing.
“Text is hot right now,” Shearer said. “A lot of companies collect text but don’t know what to do with it. Mostly, it just sits there — but, especially when it is combined with other data points, it can reveal a great deal.”
He provided an example of how it works. Say a wireless provider receives the following e-mail:
“I really feel I have to write to you about my experience since becoming your customer. As I said when I called the service center — several times, as your records will no doubt show — I am really not happy with the quality of service since I signed with you and got my new cell phone. Your advertising says you have a reputation for good coverage and high reliability, but I seem to get far more dropped calls than I used to with Yellow Inc., and I have to say I am very tempted to switch to them. But I would like to mention, I found your service center agents very helpful, with an excellent attitude. Is there some way you can help improve things for me? Please let me know.”
The terms extracted by a text mining analysis include these:
- service center
- several times
- quality of service
- new cell phone
- good coverage
- high reliability
- new cell phone
- dropped calls
- not happy
- switch to
- Yellow Inc.
- service center agents
- very helpful
- excellent attitude
An analysis of the categories impacted by this customer’s e-mail includes these observations:
- Quality of service [Negative]
- Service center [Positive]
- Cell phone [Negative]
- Competition [Yellow Inc.]
The result, ideally, is that the company will respond to the customer’s specific complaints instead of dispatching a bland letter indicating awareness of trouble, etc. Because the customer is happy with other aspects of the service, such an overture would be likely to head off a departure.
“It is how BI was intended to be used all along,” Shearer said. “Identify a potential trouble spot, giving the company the time and information it needs to head off customer defection.”
This technology has been around for a few years — largely as an experimental or niche offering, but it is becoming more mainstream, according to Shearer, as more companies include e-mail responses in their customer service channels.
Managing Databases
While text is indeed becoming a channel of choice for many consumers, it is still one channel. No company can afford to ignore voice, for instance, or to ignore the many other databases in which customer data resides.
Integrating multiple sources of data has been one particularly difficult challenge for BI applications, according to Roland Markowski, managing director ofSAND Central Europe.
One of the firm’s largest customers, AOL Germany, came to SAND for help, he told CRM Buyer. It wanted to capture customer data — such as level of profitability, loyalty profiles and use of the product — from multiple sources, to prepare the data for analytic use, and make it accessible to support decision-making.
SAND has developed a favorable reputation among ISPs, with three of the top five vendors using its technology, according to the firm. “This is a highly competitive environment, and once they get a customer, they want to keep that customer,” Markowski said. “So they need to analyze a high volume of data each day to meet that goal.”
There are several challenges, though. It is difficult to get a holistic view of a customer from disparate databases, for starters. If a marketing analyst wants to develop a custom campaign aimed at a specific profile — for instance, 30 to 35 year old females who are married with children, with a specific income, who have purchased X product and are AOL customers — it can take days to receive the answer, he said.
Once they do have an answer, more time might be needed to tweak the attributes to customize the campaign or arrive at just the right profile.
SAND’s databases can churn out this information, though, much more quickly, Markowski explained, for a number of reasons — including the speed of its analytics engine and its lack of indexing requirements. Because the technology is “index-free,” data can be accessed immediately after loading, and queries can be quickly altered.
Getting back to AOL, it had realized that its database marketing department was spending about 80 percent of its time just integrating data from various systems and only 20 percent of its time on actual analysis.
Using SAND, it decided to create a warehouse that would periodically collect data from various sources and make current information available in real-time via a single centralized system.
Social CRM
See all Social CRM