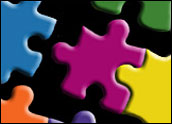
The customer is king again. After decades of mass-market driven customer outreach, which typically lumped customers in large, homogenous segments, a complex mix of socioeconomic factors coupled with a proliferation of new media and technologies have put the customer back in the driver’s seat.
Today’s customers perceive themselves as having unique needs and interests. The challenge to businesses is that, short of employing a psychic, it’s difficult to know what’s on your customers’ minds today, much less anticipate what they may need or want tomorrow.
The challenge isn’t a lack of customer data. In fact, customers leave clues about themselves in every response, contact, event, transaction and Web site hit, as well as in their failure to make a purchase or respond to a particular offer.
Databases and CRM systems are chock full of these useful tidbits. Raw data, however, does not have value per se until it is turned into information that is readily available for decision support. This is where analytics, and particularly analytical CRM, comes into play.
Meaningful Patterns
Traditional analytics, often found in reporting and business intelligence solutions, provides historical analysis, summarizing what happened in the past. In other words, historical analysis reveals what a customer bought last month or last week.
While this kind of traditional analysis is important, it doesn’t anticipate what customers will do in the future. In order to make effective decisions, organizations need to complement traditional analytics with predictive analytics, which provide a clear picture of what is going to happen.
The predictive analytic process enables companies to discover meaningful patterns and relationships in data — separating signals from noise — and provides decision-making information about the future. Companies learn, for example, what a specific customer will buy next, or which customers are likely to defect. This forward-facing customer insight enables businesses to be proactive, rather than reactive.
Predictive analytic solutions apply sophisticated statistical and machine-learning techniques to data residing in CRM and transactional systems, structured databases and flat files. Organizations also use predictive analytics to analyze Web logs and textual data, such as notes fields from sales force automation and call center applications. By supporting CRM with predictive analytics, companies of varying sizes are able to manage customer information as a strategic asset.
Three Techniques
Data mining, text mining and predictive Web analytics are three examples of predictive analytic techniques, and are described below:
Data mining procedures include: association, looking for patterns in which one event is connected to another; sequence or path analysis, looking for patterns where one event leads to later event; classification, looking for new patterns; clustering, finding and visually documenting groups of facts not previously known; and forecasting, discovering patterns in data that can lead to reasonable predictions about the future.
By using data mining, one U.S.-based telecommunications provider decreased its average monthly churn rate by as much as 25 percent while reducing the cost of its marketing efforts by 60 percent.
Text mining extracts terms and phrases and automatically classifies them into related groups, such as products, organizations or people, using the meaning and context of the text.
Coordinating Techniques
Many companies combine this distilled information with other data sources and use text mining in coordination with techniques such as clustering, classification and predictive modeling.
For example, a U.S. company uses text mining to sort and route customer comments from a national network of service centers to the appropriate individuals in the organization, providing valuable information to its product developers while increasing customer satisfaction.
Predictive Web analytics’ four analytical capabilities help organizations: segment visitors based on their behavior, detect content and product affinities, automatic identify the most significant paths taken through a Web site, and predict visitors’ propensity — for example, to make a purchase, view particular content, or churn.
A personal computer and software reseller based in Japan used predictive Web analytics to build a recommendation engine that suggests appropriate products to its Web site visitors based on their personal profiles. This has resulted in a 67 percent increase in average monthly page views, an 18 percent growth in sales, and a 200 percent gain in profits.
More Scientific Approach
With the massive amounts of customer data generated every minute, and the absolute necessity of managing each customer relationship by understanding current needs and anticipating future needs, predictive analytics is no longer a nice thing to have; it is essential.
Today’s customers want to feel unique and recognized, and they are forcing a more scientific approach to CRM. Businesses must take the time — and use the right tools — to truly understand and satisfy each customer, today and in the future.
Colin Shearer is vice president of customer analytics for SPSS Inc.