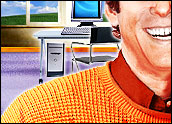
Now that e-commerce is no longer a novelty, most corporations recognize some basic realities about online sales: Building and maintaining a Web site is no walk in the park; not all browsers are equally interested in buying; and when it comes to sales, slick Web copy can’t replace the human touch.
There’s not much to be done about the first two realities, but forward-thinking corporations are addressing the third by deploying talented sales teams to the virtual storefront. The opportunity to interact with a salesperson in real time is often the critical factor in an undecided browser’s conversion into actual customer.
That said, for companies whose sites receive hundreds or thousands of hits per day, it’s simply not cost-effective — even if it were possible — to interact personally with every browser. As a result, sales reps spend a lot of time chatting with visitors who are less prepared or able to complete a transaction.
In traditional sales, the sales rep can depend on voice inflection, eye contact or body language to judge which potential customers are most interested in buying. But in the disembodied world of the Internet, such clues are entirely absent. How, then, can sales reps determine which browsers are most likely to welcome a chat?
New Rules, New Environment
One solution is for the company to create a set of business rules against which a visitor’s site activity is measured. For instance, visitors who stay on a site longer than five minutes and who look at the online application might be flagged as likely candidates for a chat. Such a rule makes sense — but it’s based on pure speculation.
What if, in reality, most visitors who stay for over five minutes are simply gathering information? What if a customer who visits for short periods over multiple days is actually the more likely candidate for immediate conversion? The truth is that no matter how detailed rules become, they are still based on intuition rather than solid evidence.
The process of targeting visitors for personal interaction improves when human guesswork is removed. We recently conducted an experiment that proved this to be the case.Removing the GuessworkTo determine which site visitors are most likely to convert, companies first need to find out which kinds of browser activities actually precede each conversion (not what they think those activities might be). We did this by collecting raw visitor activity data for 90 days from the Web site of a financial services corporation we’ll call ABC Company.
During this period, our data mining engine tracked the activities of all site visitors, while the sales server randomly designated which visitors would be targeted for a chat with a sales rep. When the data collection period was over, our scoring engine analyzed visitor activities that led to each conversion. (What windows did they open? How long did they view each page? How many times did they visit the site before converting?)
Based on this regression analysis, we created a model, which represented the ideal visitor — the one most likely to convert. Our scoring engine could then give new Web site visitors a score representing how closely their on-line actions conformed to the ideal. The higher the visitor scored, the greater the statistical likelihood that the visitor would convert. To test our theory, we needed to see what percentage of the visitors flagged as good conversion candidates actually went on to convert.
Testing the Theory
After the initial 90-day collection period, we ran the experiment again for over 32,000 approached visitors, to see whether visitors whose scores most closely matched the ideal would indeed have a higher conversion rate. According to the statistical principle of normal distribution, we expected most visitors’ scores to be close to the average, while fewer would be extremely low or extremely high. We then looked at how this distribution compared to conversion rates.
Figure 1 shows the actual number of visitors to Company ABC’s site, and their conversion rate distribution using the principle of standard deviation. (A standard deviation is how closely the various examples are to average in a set of data.)
Company ABCStandard DeviationVisitorsConversion Rate-31,64712%-210,66418%113,30632%24,25837%31,63342%Total31,96527%
Note that 42 percent of visitors who were three standard deviations above average completed the application, while only 12 percent of those three standard deviations below average did so. This chart shows that as scores got larger, conversion rates followed.
Applying the Results
The results of this experiment were significant because they demonstrate that intuition can be replaced by quantifiable data about which visitor activities indicate strongest interest; and the irreplaceable human touch (a precious and limited resource) can be reserved for site visitors most likely to become customers.
ABC Company now uses the scoring engine to rank all current visitors. Sales reps never have to review visitor data or guess who to chat with. Instead, once the engine is turned on, the sales server uses the visitor scores to determine who should receive an invitation to chat. This method takes the guesswork out of the equation, allowing ABC Company — and any company that follows this approach — to make better use of its talented sales people in the e-commerce channel.
This kind of data collection and analysis ensures that the company’s sales team can spend time with the likeliest prospects, thus improving sales, as well as the customer experience.
Vikas Rijsinghani is the Chief Technology Officer for Proficient Systems in Atlanta. He can be reached at [email protected].
Social CRM
See all Social CRM