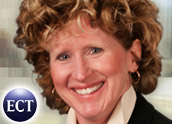
These are exciting times to be a data analyst. I say this because two of the biggest barriers that have traditionally prevented analysts from effectively leveraging and acting upon data have been greatly reduced.
The time required to compile and process data to make it useful for analysis (which can be up to 75-80 percent of the total time requirements for analytical projects), plus our ability to act on the results of data analysis in a timely and practical manner have been greatly improved by database services and technology.
In addition, research is increasingly demonstrating that marketers who employ advanced data analysis and multivariate dynamic targeting are experiencing conversions significantly above industry averages. These studies provide additional impetus to leverage data in meaningful and practical ways. Yet less than one half of companies use anything beyond the most basic testing or personalization. Why is this?
Establishing a Framework
There are many reasons, but I believe that one of the biggest barriers to fully leveraging the value of data is that, since there’s so much of it available to us, we don’t know what questions to ask of it — or how to use the results effectively. Because of the volume and complexity, I find that one of the most essential steps to a solution is to build frameworks that simplify things.
One valuable framework involves a way of looking at data stages and processes to allow us to identify obstacles to effective data use. There are often notable gaps in the data flow — data has its own life cycle, and there are stages in that life cycle where things break down and opportunities are lost.
Stage 1: Data Aggregation (getting data together). Data aggregation is a combination of collection, integration and understanding. This initial stage is probably the most important, and not only because it kicks off the cycle. This stage also entails getting familiar with your data and developing an understanding that maps data and analyses to business and marketing objectives. It sets the foundation for everything that follows; this is where you:
- Identify your business/marketing objectives in the context of strategic initiatives
- Translate them into measurable terms (a process we call “operationalizing objectives”)
- Determine the data elements required and available to address these operationalized objectives
- Perform gaps analyses where data are not readily available, and opportunities assessment where data can be leveraged
Of course there are frameworks and procedures for facilitating and simplifying this process as well. Strategic initiatives for most direct marketing campaigns fall under one of four general categories: retention/loyalty, acquisition/referrals, revenue increases (including upsell/cross-sell), and cost-savings. Specific business or marketing objectives are aligned with these initiatives, and tactics are designed to elicit the desired consumer behaviors in line with the objectives. Therefore, devoting the appropriate amount of time and energy to developing an understanding of the collected and integrated data is critical: Paying lip service can only mean headache down the road. Whether on a micro/campaign level or a macro/program level, stage one is very important.
Stage 2: Augmentation (filling in the gaps). Data augmentation usually comes in the form of primary research (surveys and focus groups) or data overlays (lifestyle and psychographic). But data augmentation can also mean integrating additional response and classification data as well — depending on your current systems. Based on the results of your gaps analysis and opportunities assessment from stage one, the efforts of augmentation are intended to fill in those gaps or perhaps create derived elements to capitalize on opportunities.
The principal value in this data stage is often the generation of behavioral narratives and profiles to better understand your customer. This is where the creative genius comes in to interpret the profiles and develop messaging and content that is appropriate for the targeted consumer audience. Aligning tone and offers with relevant consumer profiles goes a long way towards creating the trust necessary for long-term relationships.
Stage 3: Processing (making data useful). The database services that collect and integrate data typically take care of the standardization, normalization, hygiene, variable transformation functions, etc. that used to take up so much of an analyst’s time. But there is still a tremendous amount of data untapped.We have traditionally relied on structured data — that is, data found in relational databases, rows and columns — for analyses. We can do some very meaningful analyses with structured data, but it is only part of the picture. We can also analyze patterns in unstructured data, such as contained in documents, HTML templates and program logic. Companies are now starting to analyze unstructured and structured data together, with very powerful results. But first the unstructured data must be processed and translated to render it useful.
Stage 4: Analysis (making data meaningful). This is where the fun begins for most analysts. It is also the easy part. Given a set of data with its unique characteristics (that we are now very familiar with as a result of our stage one efforts), and specific analysis objectives (the “operationalized business objectives” also described in stage one), the particular statistical approach is fairly predetermined.
To further simplify things, there are five objectives served by statistical analysis: Description (distributions and central tendencies), segmentation (grouping similar things together), prediction/targeting (selecting customers for special treatment), tracking (including ROI models as well as most testing methodologies) and data mining (looking for patterns). These analysis objectives employ techniques with fancy names — like “logic regression” for prediction or “density linkage cluster analyses” for segmentation — but the purposes for which employed are relatively simple and straightforward.
Stage 5: Execution (acting on data). You have more data; now what? If you can’t make sense of it, or act on it, then it’s all so very esoteric. Another place where things frequently break down in the effective use of data is in execution: How do you leverage the results of analyses in tactical communications programs in ways that are practical and timely?
Again, it is critical to simplify: Start small and iterate. Build in some basic business rules that are refined over time. These business rules are a simply a framework for applying the output from analytics that are continuously evolved. A basic example can be stated as “If segment ‘X’ and purchase from product group ‘Y’ and target score is greater than ‘Z’ then …”
Asking the Right Questions
With all the data available to us, the challenge is in deciding what questions to ask of the data from among so many possible ones. How can you determine priorities based on what’s realistic and actionable?
By viewing data and analysis in stages, diagnostics and planning are simplified; insights are provided into where in the cycle problems or roadblocks arise, as well as what solutions are likely to be effective.
Katie Cole is vice president of analytics and research for Quris, a customer-centric email solutions agency forFortune 1000 companies. Cole has over 20 years of in-depth knowledge and experiencein data mining, statistical modeling, primary research and softwaredevelopment in a variety of industries including telecommunications,broadband, Internet and financial services.
Social CRM
See all Social CRM